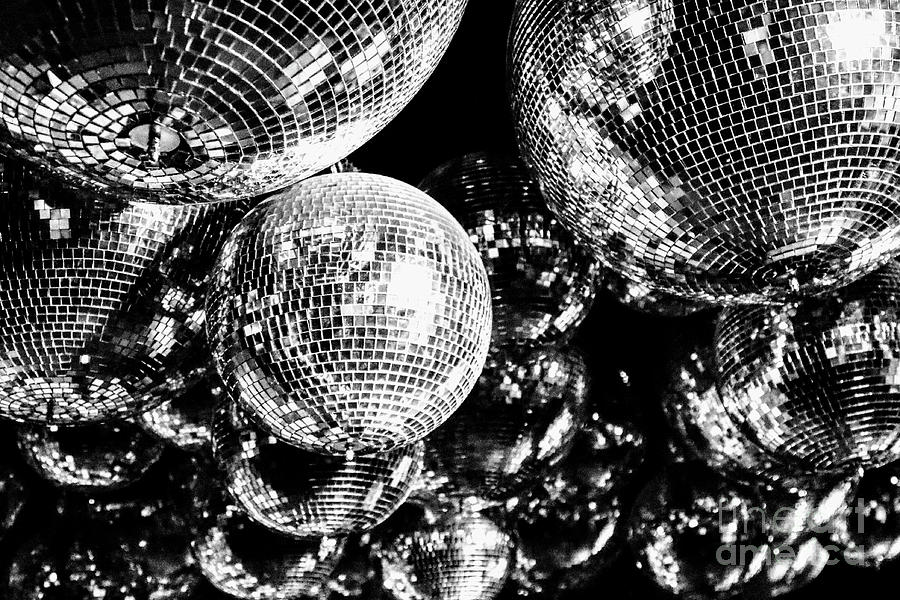
https://www.wcvb.com/article/several-injured-in-escalator-malfunction-at-boston-t-station/37746010#
Newlyweds say, 'I do,' on US-Canada border, so bride's family can attend
https://www.wcvb.com/article/newlyweds-say-i-do-on-us-canada-border-so-brides-family-can-attend/37736976

Why Now Is the Time to Jump on a New Home
https://www.htvnativeadsolutions.com/wcvb/sponsoredarticles/adv/?prx_t=LZcGA9J5LAqMcQA&ntv_acpl=1099688&ntv_acsc=1&qls=NAB_142779059.298449283&brandcontent=&dclid=CJ3vnOe8nvMCFUJBDQod6w8DxA

Kids raise money for hospital workers who care for COVID-19 patients
https://www.wcvb.com/article/kids-raise-money-for-hospital-workers-who-care-for-covid-19-patients/37735392
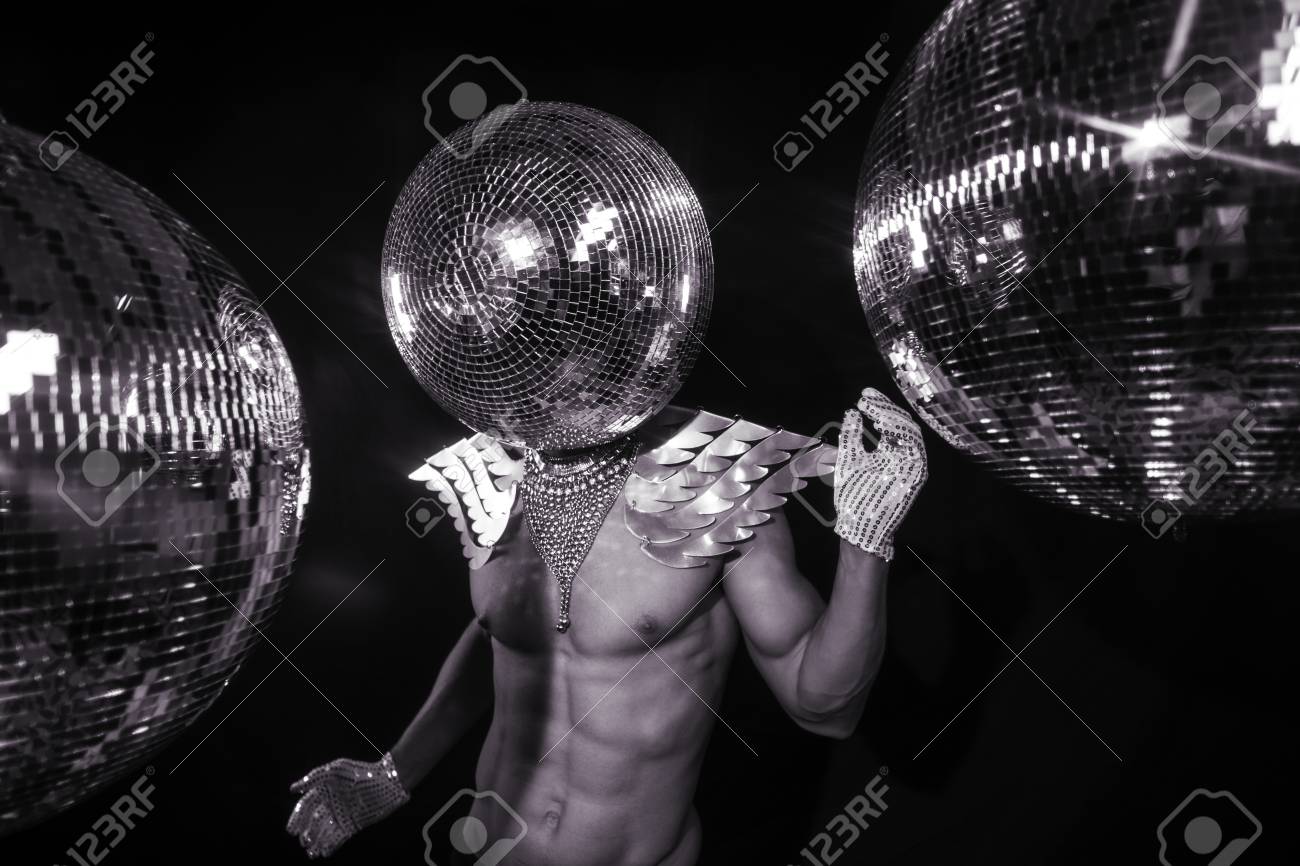
'Never thought it could happen to me': Bone marrow transplant saves mother battling leukemia
https://www.wcvb.com/article/bone-marrow-transplant-saves-life-murphys-mother-battling-leukemia/37734651

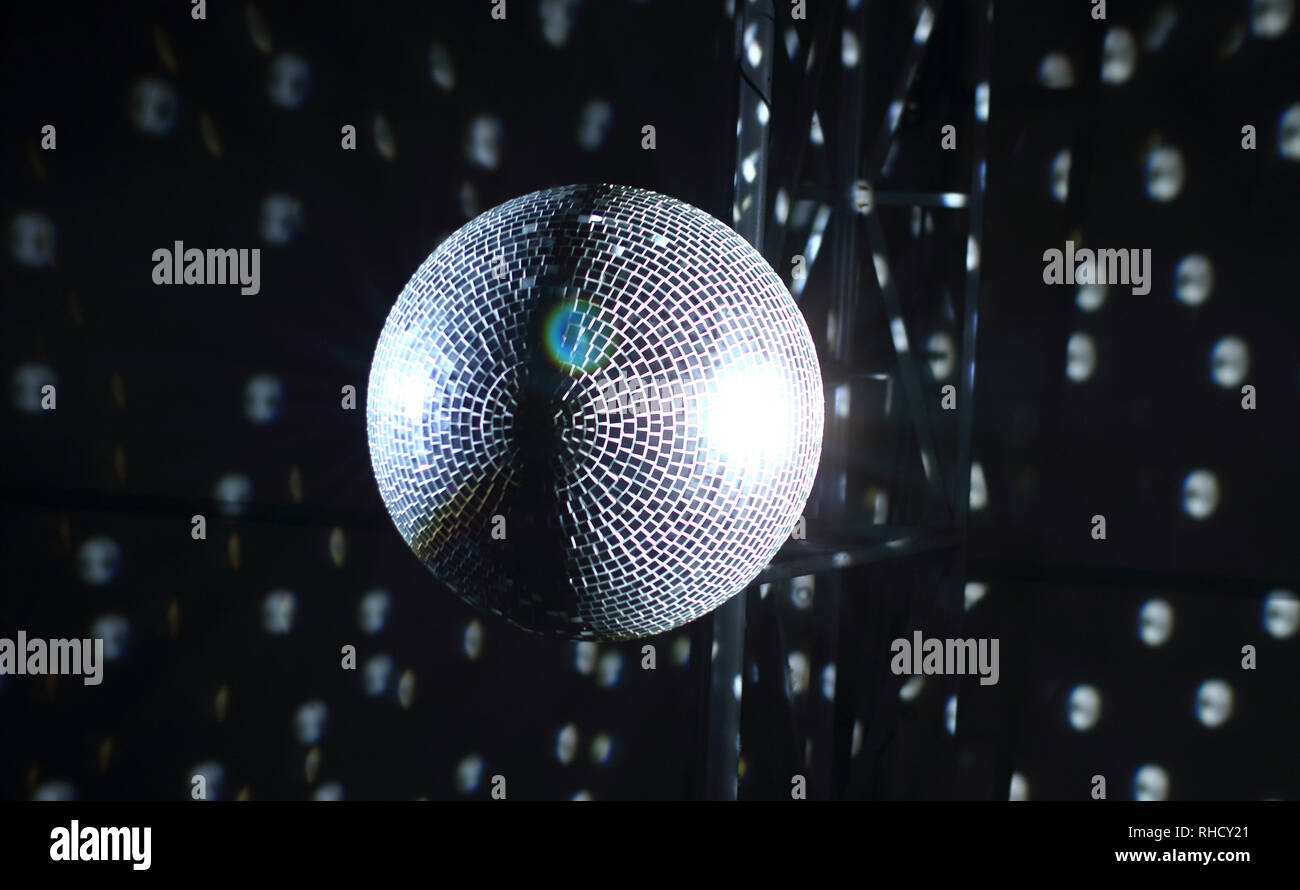

Queen – Bohemian Rhapsody (Official Video Remastered)
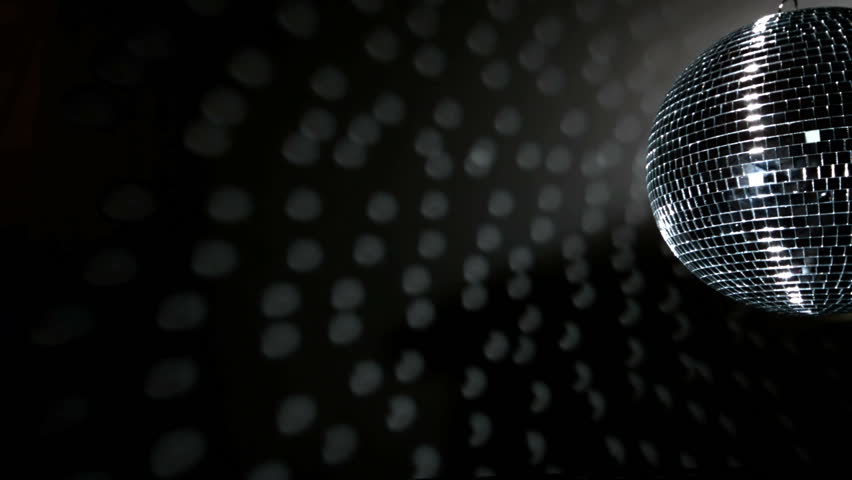
In Euclidean geometry, uniform scaling (or isotropic scaling[1]) is a linear transformation that enlarges (increases) or shrinks (diminishes) objects by a scale factor that is the same in all directions. The result of uniform scaling is similar (in the geometric sense) to the original. A scale factor of 1 is normally allowed, so that congruent shapes are also classed as similar. Uniform scaling happens, for example, when enlarging or reducing a photograph, or when creating a scale model of a building, car, airplane, etc.
More general is scaling with a separate scale factor for each axis direction. Non-uniform scaling (anisotropic scaling) is obtained when at least one of the scaling factors is different from the others; a special case is directional scaling or stretching (in one direction). Non-uniform scaling changes the shape of the object; e.g. a square may change into a rectangle, or into a parallelogram if the sides of the square are not parallel to the scaling axes (the angles between lines parallel to the axes are preserved, but not all angles). It occurs, for example, when a faraway billboard is viewed from an oblique angle, or when the shadow of a flat object falls on a surface that is not parallel to it.
When the scale factor is larger than 1, (uniform or non-uniform) scaling is sometimes also called dilation or enlargement. When the scale factor is a positive number smaller than 1, scaling is sometimes also called contraction.
In the most general sense, a scaling includes the case in which the directions of scaling are not perpendicular. It also includes the case in which one or more scale factors are equal to zero (projection), and the case of one or more negative scale factors (a directional scaling by -1 is equivalent to a reflection).
Scaling is a linear transformation, and a special case of homothetic transformation. In most cases, the homothetic transformations are non-linear transformations.
https://en.wikipedia.org/wiki/Scaling_(geometry)
Zero norm[edit]
In probability and functional analysis, the zero norm induces a complete metric topology for the space of measurable functions and for the F-spaceof sequences with F–norm [11] Here we mean by F-norm some real-valued function on an F-space with distance d, such that The F-norm described above is not a norm in the usual sense because it lacks the required homogeneity property.
Hamming distance of a vector from zero[edit]
In metric geometry, the discrete metric takes the value one for distinct points and zero otherwise. When applied coordinate-wise to the elements of a vector space, the discrete distance defines the Hamming distance, which is important in coding and information theory. In the field of real or complex numbers, the distance of the discrete metric from zero is not homogeneous in the non-zero point; indeed, the distance from zero remains one as its non-zero argument approaches zero. However, the discrete distance of a number from zero does satisfy the other properties of a norm, namely the triangle inequality and positive definiteness. When applied component-wise to vectors, the discrete distance from zero behaves like a non-homogeneous "norm", which counts the number of non-zero components in its vector argument; again, this non-homogeneous "norm" is discontinuous.
In signal processing and statistics, David Donoho referred to the zero "norm" with quotation marks. Following Donoho's notation, the zero "norm" of x is simply the number of non-zero coordinates of x, or the Hamming distance of the vector from zero. When this "norm" is localized to a bounded set, it is the limit of p-norms as p approaches 0. Of course, the zero "norm" is not truly a norm, because it is not positive homogeneous. Indeed, it is not even an F-norm in the sense described above, since it is discontinuous, jointly and severally, with respect to the scalar argument in scalar–vector multiplication and with respect to its vector argument. Abusing terminology, some engineers[who?] omit Donoho's quotation marks and inappropriately call the number-of-nonzeros function the L0 norm, echoing the notation for the Lebesgue space of measurable functions.
https://en.wikipedia.org/wiki/Norm_(mathematics)#Zero_norm
In mathematics, a reflection (also spelled reflexion)[1] is a mapping from a Euclidean space to itself that is an isometry with a hyperplane as a set of fixed points; this set is called the axis (in dimension 2) or plane (in dimension 3) of reflection. The image of a figure by a reflection is its mirror image in the axis or plane of reflection. For example the mirror image of the small Latin letter p for a reflection with respect to a vertical axis would look like q. Its image by reflection in a horizontal axis would look like b. A reflection is an involution: when applied twice in succession, every point returns to its original location, and every geometrical object is restored to its original state.
The term reflection is sometimes used for a larger class of mappings from a Euclidean space to itself, namely the non-identity isometries that are involutions. Such isometries have a set of fixed points (the "mirror") that is an affine subspace, but is possibly smaller than a hyperplane. For instance a reflection through a point is an involutive isometry with just one fixed point; the image of the letter p under it would look like a d. This operation is also known as a central inversion (Coxeter 1969, §7.2), and exhibits Euclidean space as a symmetric space. In a Euclidean vector space, the reflection in the point situated at the origin is the same as vector negation. Other examples include reflections in a line in three-dimensional space. Typically, however, unqualified use of the term "reflection" means reflection in a hyperplane.
A figure that does not change upon undergoing a reflection is said to have reflectional symmetry.
Some mathematicians use "flip" as a synonym for "reflection".[2][3][4]
https://en.wikipedia.org/wiki/Reflection_(mathematics)
Quaternion-derived rotation matrix[edit]
A quaternion rotation (with ) can be algebraically manipulated into a matrix rotation , where is the rotation matrix given by:[6]
Here and if q is a unit quaternion, .
This can be obtained by using vector calculus and linear algebra if we express and as scalar and vector parts and use the formula for the multiplication operation in the equation . If we write as , as and as , where , our equation turns into . By using the formula for multiplication of two quaternions that are expressed as scalar and vector parts,
this equation can be rewritten as
where denotes the outer product, is the identity matrix and is the transformation matrix that when multiplied from the right with a vector gives the cross product .
Since , we can identify as , which upon expansion should result in the expression written in matrix form above.
https://en.wikipedia.org/wiki/Quaternions_and_spatial_rotation#Quaternion-derived_rotation_matrix
In probability theory and statistics, two real-valued random variables, , , are said to be uncorrelated if their covariance, , is zero. If two variables are uncorrelated, there is no linear relationship between them.
Uncorrelated random variables have a Pearson correlation coefficient of zero, except in the trivial case when either variable has zero variance (is a constant). In this case the correlation is undefined.
In general, uncorrelatedness is not the same as orthogonality, except in the special case where at least one of the two random variables has an expected value of 0. In this case, the covariance is the expectation of the product, and and are uncorrelated if and only if .
If and are independent, with finite second moments, then they are uncorrelated. However, not all uncorrelated variables are independent.[1]: p. 155
https://en.wikipedia.org/wiki/Uncorrelatedness_(probability_theory)
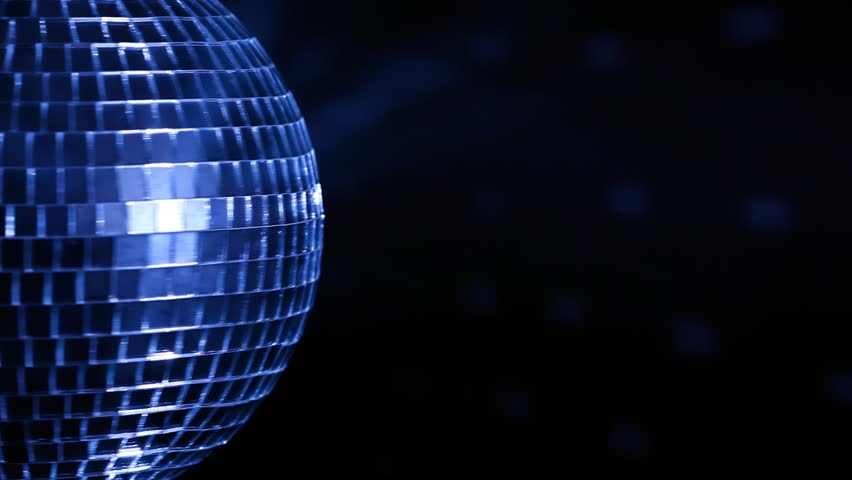
Triangle Matrix Zero
Zero Diagonal
https://en.wikipedia.org/wiki/Quaternions_and_spatial_rotation
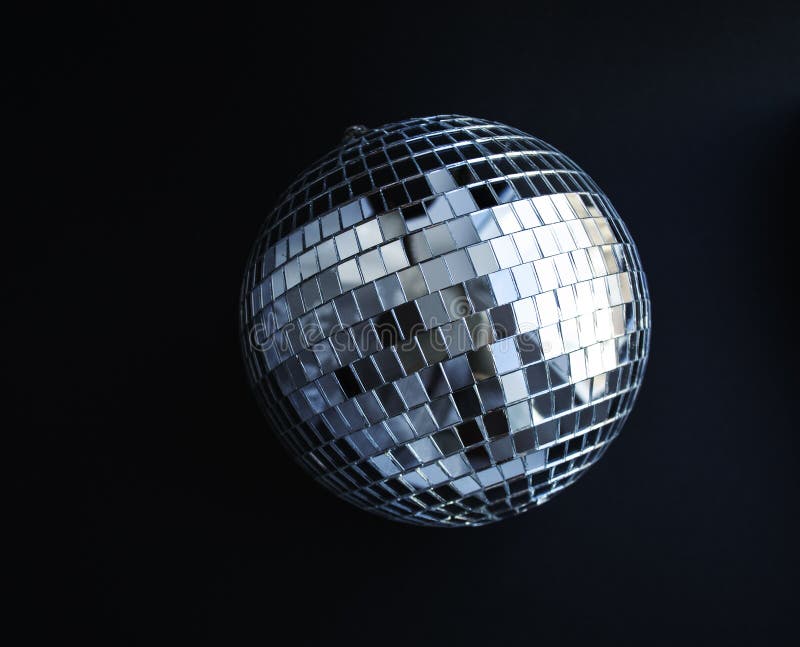
Sunday, September 26, 2021
09-25-2021-2039 - triangular matrix zero
In the mathematical discipline of linear algebra, a triangular matrix is a special kind of square matrix. A square matrix is called lower triangular if all the entries above the main diagonal are zero. Similarly, a square matrix is called upper triangular if all the entries below the main diagonal are zero.
Because matrix equations with triangular matrices are easier to solve, they are very important in numerical analysis. By the LU decomposition algorithm, an invertible matrix may be written as the product of a lower triangular matrix L and an upper triangular matrix U if and only if all its leading principal minors are non-zero.
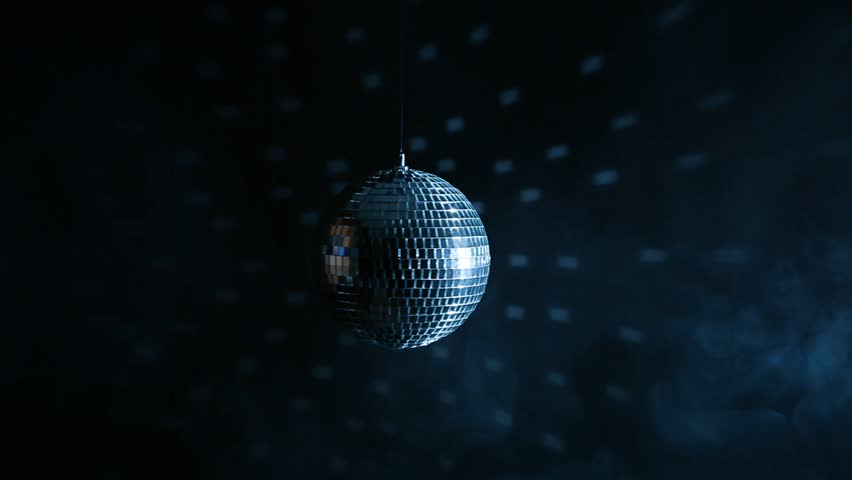
No comments:
Post a Comment